The vastly expanding world of artificial intelligence (AI) is simply difficult to comprehend. It is moving and evolving so quickly that even those who are AI professionals have difficulty keeping up with what game-changing innovations have been developed each week. This extreme speed of advancement is psychologically foreign to our human brains. We understand linear growth, linear improvement, linear aging.
The growth of AI, like other technologies of the last few decades, is not linear, it is exponential. We have difficulty seeing, for example, that it takes five years of development to reach a certain milestone. But then it only takes a single year to double that improvement. Essentially, we are taking part in pushing a technology forward, in a way that is so incredibly fast that we can intuitively gauge its speed.
In the case of AI, this is also hampered by the fact that most people simply don’t understand how AI works: how it is developed, how it is coded, how it is trained, and how it “thinks”. This creates yet more complications when trying to build up a system that can effectively create AI, especially AI models significantly larger than what we have today.
A normal development process would involve various companies competing to make more and more capable tools. In some ways, AI has done this up to a point. Many different companies have made tools that either allow people to use AI, or allow them to build AI models of their own. In either case, these companies have invested heavily into the capital needed for the software and especially the hardware to create these innovations.
Even the use of cloud infrastructure is still finite, with companies renting a certain amount of processing and storage. The problem is, with exponential development this process becomes increasingly difficult for a single company to bear the burden. For example, Google is investing $10 billion in OpenAI’s products. In a few years, even this won’t be enough.
The fact is, exponential growth can’t follow the traditional model. If AI is to continue growing at this rate, it needs a different type of infrastructure to handle it. And this is where a decentralized AI model is emerging. The decentralized architecture certainly isn’t new, but it has been supercharged with the emergence of blockchain helping to coordinate and scale its growth. The parallel growth of AI and blockchain is likely a coincidence, but the implications and opportunities are not.
The decentralization of AI is the natural consequence of combining the two, and it is bound to help AI continue on its exponential path. A number of key leaders in the field have seen this as well, with And combined, with Stability AI’s CEO Emad Mostaque stepping down and stating that you can’t “beat centralized AI with more centralized AI”. A report by TenSquared Capital discusses how AI’s growth is firmly rooted in blockchain capabilities. The bottom line is, there are three key reasons why decentralized AI will outshine centralized AI without question.
Scalability
If the past few years are any indication (and they are), AI’s biggest constraint will be scalability. As AI continues to develop new capabilities, it comes with a massive appetite for training data and model learning/development. Running an algorithm, especially a Large Language Model (LLM) like ChatGPT, requires unbelievable hardware requirements. But as big as ChatGPT is, it is likely small compared to the models that will be developed and trained in just a few years.
The more data we create and collect, the more sophisticated AI models will become. And the more efficient and general-use AI models become, the more demand there will be for them. To truly support this, any AI scaling effort has to be decentralized.
Unlike some scaling solutions, AI requires both software and hardware to scale. While decentralized processing has been around for decades (the SETI program utilized volunteer PC processing power), AI brings new challenges.
First, the type of cooperation required for an AI model is tremendously more complex that simple processing. Parallel computing needs to be parsed out precisely, then reintegrated into the model. In addition, a normal PC, laptop, and especially a phone would be limited in their capacity to run complex algorithms, so a specific type of hardware is ideal. The furthest along in this development is likely HyperCycle, which builds AI machines for users to purchase and operate, acting much like nodes in a decentralized network and sharing computational duties under the eye of an architecture that coordinates each node’s computation and the integration of the processed information.
The modular format is perfectly aligned to be indefinitely scalable, and the costs for clients using the system for their AI needs is dependent on demand and the supply offered will likely follow the supply/demand natural market model, whereas demand goes up, prices go up, incentivizing more nodes to be purchased and set up by users.
Security
Once an AI processing infrastructure becomes decentralized, it opens itself up to major security risks if not properly addressed. Here too blockchain has developed many of the tools and processes needed to keep AI models safe and secure from bad actors. The models themselves can be encrypted using a variety of techniques, the most notable is likely some form of Zero Knowledge ZK methodology.
This allows data to remain on-chain and encrypted, yet still interact with other nodes using Multi-Party Computation (MPC). This is a critical piece of decentralized AI architecture as the secure and private nature enables countless use cases, from using sensitive data (think company private, or personal health records) to developing proprietary models.
Transparency
While all decentralized AI models should be secure, some should not, in fact, be private as well. There will be many use cases where AI models serve a public good, and as a result must be trustworthy. The best solution for this is full transparency, where anyone can see what a given model is doing, the thought process, and the weights/biases used to make a particular decision. For transparency, on-chain information is the perfect solution as it can be posted and viewed in a way that is completely visible, yet the natural immutability of blockchain ensures that the data is not changed. This protects the data, as well as the general community who needs to have total clarity with certain AI use cases.
Closing Thoughts
As AI models become larger, more complex, and are involved in more parts of our lives, decentralized AI is going to be the dominant architecture for AI development, training, and deployment of models. The decentralized nature fits well with AI’s exponential growth, and platforms like HyperCycle are already developing both the hardware and software needed to deploy AI that is scalable, secure, and when needed, transparent. The combination of AI and blockchain is truly unique, and will allow our AI journey to continue growing strong into the foreseeable future.
Disclaimer: Coinspeaker is committed to providing unbiased and transparent reporting. This article aims to deliver accurate and timely information but should not be taken as financial or investment advice. Since market conditions can change rapidly, we encourage you to verify information on your own and consult with a professional before making any decisions based on this content.
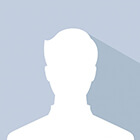
Devan is a crypto trader and Bitcoin enthusiast. He does his best to keep up to date with all the latest trends and innovations in the blockchain industry and likes sharing his expertise.